Comparison of deep learning models for weather forecasting in different climatic zones
(1) BRAC University
(2) BRAC University
(3) BRAC University
(4) BRAC University
Abstract
Keywords
Full Text:
PDFReferences
Tahsin, Mohammad Sadman, et al. "A comparative study on data mining models for weather forecasting: A case study on chittagong, Bangladesh." Natural Hazards Research (2023), ISSN 2666-5921,https://doi.org/10.1016/j.nhres.2023.12.014. (https://www.sciencedirect.com/science/article/pii/S2666592123001373)
Hill, A. J., Herman, G. R., & Schumacher, R. S. (2020). Forecasting Severe Weather with Random Forests, Monthly Weather Review, 148(5), 2135-2161. Retrieved Feb 13, 2023, from https://journals.ametsoc.org/view/journals/mwre/148/5/mwr-d-19-0344.1.xml
W. Zhang, H. Zhang, J. Liu, K. Li, D. Yang and H. Tian, "Weather prediction with multiclass support vector machines in the fault detection of photovoltaic system," in IEEE/CAA Journal of Automatica Sinica, vol. 4, no. 3, pp. 520-525, 2017, doi: 10.1109/JAS.2017.7510562.
Kartika Purwandari, Join W.C. Sigalingging, Tjeng Wawan Cenggoro, Bens Pardamean, Multi-class Weather Forecasting from Twitter Using Machine Learning Aprroaches, Procedia Computer Science, Volume 179, 2021, Pages 47-54, ISSN 1877-0509, https://doi.org/10.1016/j.procs.2020.12.006.
Rasp, S., Dueben, P. D., Scher, S., Weyn, J. A., Mouatadid, S., & Thuerey, N. (2020). WeatherBench: A benchmark data set for data-driven weather forecasting. Journal of Advances in Modeling Earth Systems, 12, e2020MS002203. https://doi.org/10.1029/2020MS002203
Datta, A., Si, S., Biswas, S. (2020). Complete Statistical Analysis to Weather Forecasting. In: Das, A., Nayak, J., Naik, B., Pati, S., Pelusi, D. (eds) Computational Intelligence in Pattern Recognition. Advances in Intelligent Systems and Computing, vol 999. Springer, Singapore. https://doi.org/10.1007/978-981-13-9042-5_65
M. S. Tahsin, M. A. Karim, M. U. Ahmed, Y. Rahman, F. Tafannum and S. Abdullah, "Comparative Analysis of Weather Prediction Using Ensemble Learning Models and Neural Network," 2021 19th OITS International Conference on Information Technology (OCIT), Bhubaneswar, India, 2021, pp. 325-330, doi: 10.1109/OCIT53463.2021.00071.
He, S., Li, X., Trenary, L., Cash, B. A., DelSole, T., & Banerjee, A. (2022). Learning and Dynamical Models for Sub-seasonal Climate Forecasting: Comparison and Collaboration. Proceedings of the AAAI Conference on Artificial Intelligence, 36(4), 4495-4503. https://doi.org/10.1609/aaai.v36i4.20372
Azam Moosavi, Vishwas Rao, Adrian Sandu, Machine learning based algorithms for uncertainty quantification in numerical weather prediction models, Journal of Computational Science, Volume 50, 2021, 101295, ISSN 1877-7503, https://doi.org/10.1016/j.jocs.2020.101295. (https://www.sciencedirect.com/science/article/pii/S1877750320305895)
Ryuji Kimura, Numerical weather prediction, Journal of Wind Engineering and Industrial Aerodynamics, Volume 90, Issues 12–15, 2002, Pages 1403-1414, ISSN 0167-6105, https://doi.org/10.1016/S0167-6105(02)00261-1. (https://www.sciencedirect.com/science/article/pii/S0167610502002611)
Donadio, L.; Fang, J.; Porté-Agel, F. Numerical Weather Prediction and Artificial Neural Network Coupling for Wind Energy Forecast. Energies 2021, 14, 338. https://doi.org/10.3390/en14020338
Mahmood, M.R., Patra, R.K., Raja, R., Sinha, G.R. (2019). A Novel Approach for Weather Prediction Using Forecasting Analysis and Data Mining Techniques. In: Saini, H., Singh, R., Kumar, G., Rather, G., Santhi, K. (eds) Innovations in Electronics and Communication Engineering. Lecture Notes in Networks and Systems, vol 65. Springer, Singapore. https://doi.org/10.1007/978-981-13-3765-9_50
Zahra Karevan, Johan A.K. Suykens, Transductive LSTM for time-series prediction: An application to weather forecasting, Neural Networks, Volume 125, 2020, Pages 1-9, ISSN 0893-6080, https://doi.org/10.1016/j.neunet.2019.12.030. (https://www.sciencedirect.com/science/article/pii/S0893608020300010)
Dimri, T., Ahmad, S. & Sharif, M. Time series analysis of climate variables using seasonal ARIMA approach. J Earth Syst Sci 129, 149 (2020). https://doi.org/10.1007/s12040-020-01408-x
Stackhouse, P. (n.d.). NASA POWER. Retrieved February 1, 2023, from Nasa.gov website: https://power.larc.nasa.gov/data-access-viewer/
Habumugisha, J.M.; Chen, N.; Rahman, M.; Islam, M.M.; Ahmad, H.; Elbeltagi, A.; Sharma, G.; Liza, S.N.; Dewan, A. Landslide Susceptibility Mapping with Deep Learning Algorithms. Sustainability 2022, 14, 1734. https://doi.org/10.3390/su14031734
Rafaela Castro, Yania M. Souto, Eduardo Ogasawara, Fabio Porto, Eduardo Bezerra, STConvS2S: Spatiotemporal Convolutional Sequence to Sequence Network for weather forecasting, Neurocomputing, Volume 426, 2021, Pages 285-298, ISSN 0925-2312, https://doi.org/10.1016/j.neucom.2020.09.060. (https://www.sciencedirect.com/science/article/pii/S0925231220315794)
Wu, Z., et al.: A comprehensive review on deep learning approaches in wind forecasting applications. CAAI Trans. Intell. Technol. 7( 2), 129– 143 (2022). https://doi.org/10.1049/cit2.12076
Refbacks
- There are currently no refbacks.
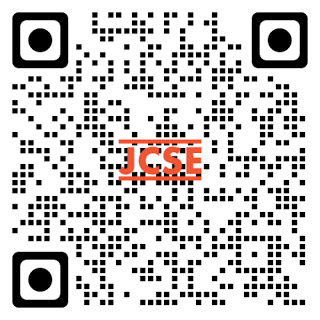
Published by : ICSE (Institute of Computer Sciences and Engineering)
Website : http://icsejournal.com/index.php/JCSE/
Email: jcse@icsejournal.com
is licensed under a Creative Commons Attribution-ShareAlike 4.0 International License.