Forecasting Indonesia's Unemployment Rates Using Moving Average Methods
(1) Institut Teknologi Nasional Malang
(2) Institut Teknologi Nasional Malang)
Abstract
Keywords
Full Text:
PDFReferences
E. Kurniawan A., M. Awaluddin, F. Fitriadi, A. Busari, and D. C. Darma, “Contemporary Indonesian GDP: Context of Analysis at Unemployment, Labor Force and Poor People,” International Journal of Economics and Financial Research, no. 74. Academic Research Publishing Group (Publications), pp. 143–154, Nov. 25, 2021. doi: 10.32861/ijefr.74.143.154
Fatkhu Rokhim, “Factors Influencing Unemployment in Indonesia,” Journal of Scientific Research, Education, and Technology (JSRET), vol. 2, no. 1. Kirana Publisher, pp. 122–131, Jan. 31, 2023. doi: 10.58526/jsret.v2i1.51
E. A. R. Puspadjuita, “Factors that Influence the Rate of Unemployment in Indonesia,” International Journal of Economics and Finance, vol. 10, no. 1. Canadian Center of Science and Education, p. 140, Dec. 10, 2017. doi: 10.5539/ijef.v10n1p140.
P. Eko Prasetyo, “The Role of MSME on Unemployment in Indonesia”, TURCOMAT, vol. 12, no. 13, pp. 2519–2525, Jun. 2021. doi: 10.17762/turcomat.v12i13.8944
A. Rahmawati and P. Putri, “The Effect of Education Variable on the Open Unemployment Rate”, efficient, vol. 4, no. 2, pp. 1160-1173, Jun. 2021. doi: 10.15294/efficient.v4i2.46128
E. K. Oktafianto, N. A. Achsani, and T. Irawan, “The Determinant of Regional Unemployment in Indonesia: The Spatial Durbin Models,” Signifikan: Jurnal Ilmu Ekonomi, vol. 8, no. 2. LP2M Universitas Islam Negeri (UIN) Syarif Hidayatullah Jakarta, Jul. 06, 2019. doi: 10.15408/sjie.v8i2.10124.
Fajar, M., Prasetyo, O. R., Nonalisa, S., & Wahyudi, W. Forecasting unemployment rate in the time of COVID-19 pandemic using Google trends data (case of Indonesia). 2020.
Suryadarma, D., Suryahadi, A., & Sumarto, S. The measurement and trends of unemployment in Indonesia: The issue of discouraged workers. Available at SSRN 861464. 2005.
Manurung, N. Application Of The Single Moving Average (SMA) Method For Forecasting Sales Of Horden In Umi Nala's Shop Business. In International Conference on Social, Sciences and Information Technology (Vol. 1, No. 1, pp. 297-306). 2020.
Harini, S. . Identification COVID-19 cases in Indonesia with the double exponential smoothing method. Jurnal Matematika MANTIK, 6(1), 66-75. 2020. doi: 10.15642/mantik.2020.6.1.66-75
Ning, L., Pei, L., & Li, F. Forecast of China's carbon emissions based on Arima method. Discrete Dynamics in Nature and Society, 2021, 1-12. doi:10.1155/2021/1441942
Deretić, N., Stanimirović, D., Awadh, M. A., Vujanović, N., & Djukić, A. SARIMA modelling approach for forecasting of traffic accidents. Sustainability, 14(8), 4403. 2022. doi: 10.3390/su14084403
Hari, Y., & Dewi, L. P. Forecasting system approach for stock trading with relative strength index and moving average indicator (Doctoral dissertation, Petra Christian University). 2018.
Hyndman, R. J., & Athanasopoulos, G. Forecasting: principles and practice. OTexts. 2018.
Chapman, S.N., 2006. The Fundamentals Of Production Planning And Control. [e-book] Prentice Hall : Upper Saddle River, New Jersey. Available : website < http://brharnetc.edu.in/br/wp-content/uploads/2018/11/22.pdf > [Accessed 03 Oktober 2023]
R. J. Hyndman, “Moving Averages,” International Encyclopedia of Statistical Science. Springer Berlin Heidelberg, pp. 866–869, 2011. doi: 10.1007/978-3-642-04898-2_380
Svetunkov, Ivan, and Fotios Petropoulos. "Old dog, new tricks: a modelling view of simple moving averages." International Journal of Production Research 56.18. 6034-6047. 2018. doi: 10.1080/00207543.2017.1380326
Nadhira, A. T., Gadisku, C. A., & Peranginangin, S. M. (2021). Demand Forecasting Comparison of Softex 1400-M using Single Moving Average Method and Single Exponential Smoothing Method. In Proceedings of the International Conference on Industrial Engineering and Operations Management (pp. 452-459).
Yudaruddin.R(2019). Forecasting: for economic and business activities. RV.Pustaka Horizon. ISBN:978-602-5431-48-7.
Liantoni, F., & Agusti, A. Forecasting bitcoin using double exponential smoothing method based on mean absolute percentage error. JOIV: International Journal on Informatics Visualization, 4(2), 91-95. 2020. doi: 10.30630/joiv.4.2.335
Refbacks
- There are currently no refbacks.
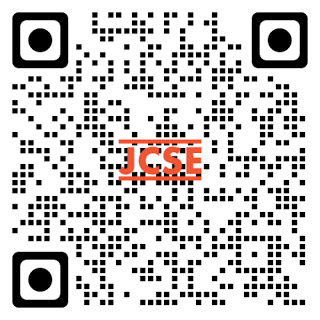
Published by : ICSE (Institute of Computer Sciences and Engineering)
Website : http://icsejournal.com/index.php/JCSE/
Email: jcse@icsejournal.com
is licensed under a Creative Commons Attribution-ShareAlike 4.0 International License.